Laboratory automation: letting scientists focus on science
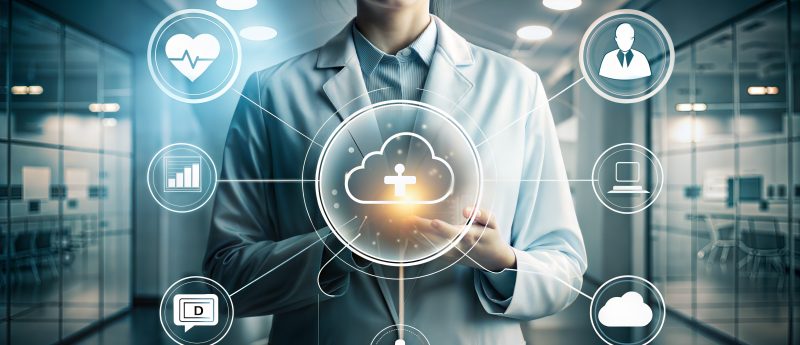
Every year, tens of thousands of young, brilliant, curious and ambitious students march into colleges with a passion for chemical and life sciences as their career choices. As the years pass by, however, the number of people who started out at the same time and manage to stick around chemical and life sciences laboratories and industries keeps dwindling. If you ask those who quit chemical and life sciences, you would often hear them say: “science is hard”.
Overcoming the reproducibility bump
Modern chemical and life sciences are hard because they are quantitative and data-centric. Long gone is the era when mere qualitative description/assessment of scientific matters would suffice. These days, a chemist or life scientist cannot draw any sound scientific conclusion without backing it up with experimental data. Often, the acquisition of trustworthy data becomes the critical bottleneck of a scientific discipline. For example, bioanalysts know full well the importance of high quality bioanalytical data to pharmacokinetics, pharmacodynamics and toxicokinetics. Without good bioanalytical data, those disciplines would not go very far.
If the life science body of knowledge were a fast moving car, then bioanalysts would be at the interface where “the rubber hits the road” – we provide the data that move life sciences ahead, and experience much friction while doing so. Specifically, getting high quality bioanalytical data is a bumpy venture if all done manually. It is not a given, not even for a GLP assay. Too often, a GLP validated bioanalytical assay fails during manual production for no apparent reason other than different bioanalysts for assay validation versus manual production. Analyst to analyst variability during manual production is actually the first major and common reason for failing a GLP assay, because no two human beings are identical to each other, not even twins. Sometimes, even a good scientist struggles to reproduce his/her own results. In a sense, one can almost say that science is hard because data reproducibility is hard to achieve with bare hands.
When I was working at the bench, manually pipetting hundreds of bioanalytical samples, I remember saying to myself: “This is not a job meant for human beings. It is a job meant for the machine.” To get quality data manually, a bioanalyst almost needs to possess a machine’s precision and reproducibility. Which is exactly the point of laboratory automation – instead of asking a human being to act like a robot in order to do a good job, why don’t we simply let the machine do the work then? Fast forward 16 years, the bioanalytical industry has now found many ways to tap into laboratory automation and overcome the reproducibility bump [1-5]. In my opinion, we need to consolidate and incarnate our collective findings into physical tools [6, 7], so that the whole bioanalytical field can move forward from an elevated platform with improved tools that embody cumulative human knowledge. Such is the true meaning of industrialization and is how many other disciplines advance. I believe the bioanalytical industry is no exception.
Accelerating scientific knowledge accumulation
The other common reason for failing a GLP validated assay manual production batch is that the method/assay was on the borderline when developed and validated to begin with. When a method is on the borderline, slight changes in laboratory environment (e.g. lighting, temperature), reagent lots, device lots (e.g. plastic pipette tips and containers), speed and duration of vortex, overall speed of the experiment, etc. could all potentially negatively affect the assay batch. A bioanalytical method is on the borderline because one or more of the key variables are not optimized during the method development (MD) process.
Bioanalytical MD, regardless of large or small molecule, drug or biomarker, is probably the most creative part of the entire bioanalytical workflow. It is but an iterative process of scientific hypothesis, experimental design, collection of quality data, cognitive scientific cerebration, revision of experimental design, and so on, so forth, until an acceptable, validate-able bioanalytical method is found. The key adjective here is ‘acceptable’ because there are unlimited permutations of experimental conditions to try – the sky is the limit, and MD scientists, especially those working in life science industries, are constantly dealing with new chemical and biological entities, and are always under pressure to rapidly deliver assays that meet regulatory requirements. As a result, assays are often times just ‘fit-for-purpose’ and not optimized strictly analytically speaking, if everything is done manually. Traditionally, the speed with which a MD bioanalyst comes up with a method depends on his/her ‘skills’ or ‘experiences’.
MD scientists in some ways are like artists – the skills are hard to develop, slow to accumulate, hard to transfer to others, and go with them. For these reasons, many bioanalytical organizations have dedicated MD groups and MD scientists, hoping to leverage the cumulative experiences and knowledge pool. It is human nature to distance ourselves from uncertainties, but MD scientists are constantly dealing with uncertainties day in day out. For that, I salute them, the heroes of bioanalytical laboratories. What is the ultimate reward for method development scientists’ jobs well done? They get promoted out of their bench positions! They can still contribute to MD through other junior scientists, but not quite as effectively as when they were at the bench, for the same reasons outlined in the previous section. Therefore, MD scientists working at the bench are often times not the most experienced ones.
If both were presented with the same, logically and chronologically organized, complete set of high quality MD data, the time it would take for a well-trained young MD scientist to reach a bioanalytical assay would not be much longer than what would take an experienced MD scientist. The point here is that well-trained scientific minds, regardless of age, work much faster than the acquisition of quality MD data, which is the bottleneck in bioanalytical MD and the accumulation of MD ‘experiences’. What some published automation work [8, 9] and ongoing research by my team on (sample preparation) MD automation systems do is to speed up the acquisition of quality MD data automatically, so that there will be more, faster and better food for thought for MD. In effect, this will speed up the accumulation of MD knowledge and experiences for MD scientists. The very same approach could be applied to essentially all bioanalytical assays [10] and incorporated into the tools mentioned above [6, 7].
When automation-assisted MD tools proliferate, will it mean that MD scientists’ experiences will be diluted and less valued? Not at all. In terms of MD experiences, what is acquired is acquired. Everybody will still be on the same level playing field. The MD automation tools would just accelerate the collection of quality MD data, and subsequently the generation of scientific knowledge. Whether experienced MD scientists choose to use these tools to continue the accumulation of MD knowledge/expertise or not would be a different story.
Conclusion
When accumulation of assay development knowledge is accelerated through laboratory automation, and rugged, laser-like precision executions of the assays are guaranteed through laboratory automation, scientists would worry less about the quality of data, spend less time on the acquisition of large amount of quality data and focus more on interpreting data and solving scientific rather than experimental problems. Scientists would work more cognitively and intellectually rather than experimentally. The composition of a bioanalyst’s work time, and more profoundly, the composition of the chemical and life science workforce itself would change gradually and accordingly. There is a glimpse of hope on the horizon that one day bioanalysts would work mostly in front of computer screens, rather than at the bench [7]. As a bioanalyst coming from the bench, I’m actually quite excited about that prospect. I hope you are, too, such that we can work together [7, 11] to make that a self-fulfilling prophecy.
References:
- Zheng N, Jiang H, Zeng J. Current advances and strategies towards fully automated sample preparation for regulated LC–MS/MS bioanalysis. Bioanalysis 6(18), 2441–2459 (2014).
- Patel V, Leach D, Hornberger M et al. Automating bioanalytical sample analysis through enhanced system integration. Bioanalysis 5(13), 1649–1659 (2013).
- Yuan L, Ji QC. Automation in new frontiers of bioanalysis: a key for quality and efficiency. Bioanalysis 4(23), 2759–2762 (2012).
- Li M, Chou J, Jing J et al. MARS: bringing the automation of small-molecule bioanalytical sample preparations to a new frontier. Bioanalysis 4(11), 1311–1326 (2012).
- Shou WZ, Zhang J. Recent development in software and automation tools for high-throughput discovery bioanalysis. Bioanalysis 4(9), 1097–1109 (2012).
- Allinson JL, Blick KE, Cohen L, Higton D, Li M. Ask the experts: automation: part I. Bioanalysis 5(16), 1953–1962 (2013).
- Li M. Automation in the bioanalytical laboratory: what is the future? Bioanalysis 5(23), 2859–2861 (2013).
- Li M, Chou J, King K, Yang L. ASPECTS: An automation-assisted SPE method development system. Bioanalysis 5(13), 1661-1676 (2013).
- Singleton C, Li M. Automated method development of sample preparation. In: Eliminating bottlenecks for efficient bioanalysis: practices and applications in drug discovery and development. Future Science Group, London, UK, 6–17 (2014).
- Li M, Chou J. Automation tools. In: E-tools in Bioanalysis. Future Science Group, London, UK (In Press) (2015).
- Li M. Bioanalytical laboratory automation development: why should we and how could we collaborate? Bioanalysis 7(2), 153-155 (2015).