AI-supported biomarker panel identifies early-stage cancers with >99% accuracy
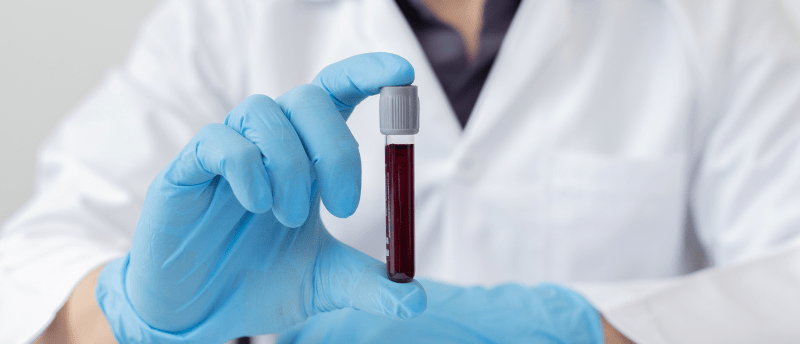
Beyond improving the likelihood of treatment success, early-stage cancer detection facilitates improved patient quality of life and significantly reduces the cost and complexity of treatment. While various screening tests exist for a confined set of cancers including breast, colorectal, cervical, lung and prostate cancers, their impact has remained sub-optimal due to their disputable efficacy and limited scope, which focuses on a single cancer type.
Although various approaches are in development, recent advancements in multi-cancer early detection (MCED) tests have shown promise for improving cancer diagnosis. These tests can be used to detect multiple cancers as they capture signals from cell-free (cf) or circulating tumor (ct) DNA or other circulating analytes that are shed by tumors into the blood. However, effective screening tests must have a high detection sensitivity and specificity and typically biomarker concentrations are low and must be distinguished from the background noise of normal human physiology.
Published in Scientific Reports, scientists from PredOmix Technologies (Gurugram, India) have developed an MCED test for women using untargeted serum metabolomics – using high-resolution mass spectrometry – combined with machine learning-powered data analytics. The team used 1926 cancer serum samples from women with either breast, endometrial, cervical, ovarian, lung, acute myeloid leukemia, thyroid, melanoma, colorectal, kidney, non-Hodgkin lymphoma, pancreatic, head and neck, gastric, liver and bile duct cancers, along with 300 samples from healthy volunteers. Positive ion mode ultra-pressure liquid chromatography coupled mass spectrometry (UPLC-MS/MS) was employed for each serum sample to provide a thorough representation of the serum metabolome. The resulting data was then resolved using two machine-learning algorithms.
- Biomarkers for oncology: an interview with Jialu Liu
- Small extracellular vesicle biomarkers in breast cancer: a real-time snapshot for early diagnosis and prognosis?
- Blood biomarkers and genomic data combined to predict disease risk
The first algorithm was used to differentiate the cancer samples from normal controls, termed the Cancer Detection Artificial Intelligence (CDAI) model. The second determined the tissue of origin (TOOAI), which layered on top of the CDAI model, acting on the cancer-positive samples to generate a multiclass score for each sample. By scoring the relative probability with which the tissue of origin of a given sample corresponded to each cancer type, the authors were able to identify the most likely tissue of origin for that sample.
The first algorithm successfully identified all the cancer-positive samples with an overall accuracy of >99% in 15 cancer types, which is particularly significant as the samples used in the study were predominantly from early-stage cancers. The authors were also able to identify the tissue of origin for a test sample group with an overall accuracy of ~92%.