Challenges with biomarker assay evaluation: endogenous analyte detectability vs assay sensitivity
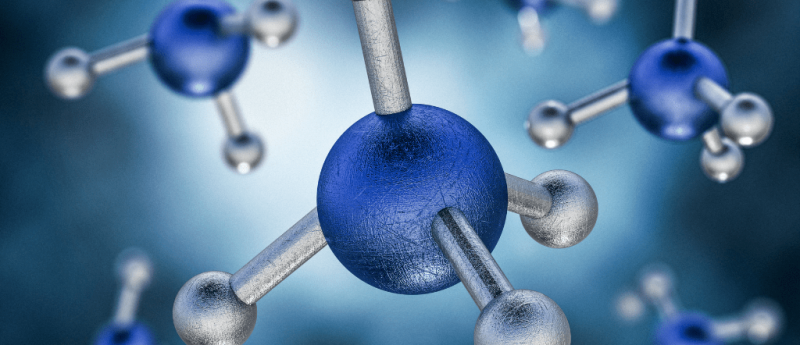
Author: Saloumeh Fischer
Author Affiliations: Genentech, Inc., South San Francisco, CA, USA
Keywords: Biomarkers, assay sensitivity, assay detectability, neurofilament light (NFL), Simoa, ELISA
Financial Disclosure: Funding for this work was provided by Genentech, Inc. SK Fischer is an employee of Genentech, Inc., and a stockholder of the Roche group.
Acknowledgements: The author would like to thank Gizette Sperinde and Dana Baker for providing the figures for this editorial.
The high rate of drug failure during clinical development has triggered an interest in using biomarkers as surrogate measures in clinical trials to optimize decision-making and resource utilization. Biomarkers are important indicators that have significant potential in therapeutic discovery, development and clinical management. From a clinical perspective, biomarker measurements are multifaceted and can be used to aid disease diagnosis (diagnostic biomarkers), determine the potential for developing a disease (susceptibility/risk), assess disease status (monitoring), evaluate target engagement (pharmacodynamics/response), predict the future course of disease (prognostic biomarkers), identify responders and non-responders (predictive and complementary biomarkers), as well as safety of the molecule. However, despite a plethora of publications regarding biomarker discovery and characterization, few useful biomarkers have been successfully validated for routine clinical use [1,2]. One reason for this limited clinical benefit is that at least 50% of published biomarker study conclusions are not reproducible [3].
When speaking about biomarkers, a distinction should be made between biomarker analytical method validation versus clinical qualification. Analytical method validation is the process of assessing the assay performance characteristics and ensuring optimal conditions for reproducibility and accuracy of the assay. Clinical qualification is the process of linking a biomarker with biological processes and clinical endpoints [4]. This editorial is focused on analytical method validation, which is the first step in obtaining meaningful results.
The quality of data generated in a study is only as good as the bioanalytical methods used. Therefore, biomarkers may fail not only because of the underlying science, but also due to poor assay choice and validation [5].
Assay selectivity/specificity
Although bioanalytical qualification/validation parameters like precision, analytical measurement ranges, parallelism and selectivity/specificity are well understood, the challenge of selecting the appropriate assays remains. This is often associated with performing the appropriate evaluation of selectivity/specificity in the correct patient population. Biomarkers assays, specifically assay kits, are often evaluated in buffer or in samples from healthy volunteers and don’t account for disease specific interference, which often exists. Matrix interference, which occurs when a nonspecific substance is present in a given sample leading to altered results, is often overlooked in assay development, which confounds discovery and development efforts [6]. These interferences are due to interactions between constituents in the sample with one or more reagent components and can be analyte dependent (caused by matrix components) or analyte-independent (caused by assay components). Assays designed to evaluate low analyte levels often detect nonspecific signals due to assay interference rather than the presence of the analyte of interest, as demonstrated previously [7]. Addressing assay interference in the appropriate matrix and patient population is important in developing an optimal biomarker assay.
Assay detectability verses assay sensitivity
Another important factor is using specific reagents that can detect endogenous analytes of interest. However, one of the challenges with biomarker assay development is that unlike a pharmacokinetic (PK) assay, where the analyte of interest is known and therefore the reagents generated are very specific to the analyte, the reagents developed for biomarkers are often generated based on full or partial length recombinant material and are not always representative of the endogenous analyte. Therefore, an assay can be very sensitive for the reagents generated based on the recombinant material used in development but fail to detect the endogenous material. This aspect of biomarker assay development highlights how using assay sensitivity to select between available assays can be misleading. Figure 1 shows a plot of different calibrators for an IL-13 assay, which are giving different assay sensitivity values despite the same capture and detection reagents used. Interestingly, a different order of assay sensitivity is achieved when different capture and detection antibodies are used. The Chinese Hamster Ovaries derived calibrator (blue dashed line) is most sensitive using the Simoa kit from Quanterix (MA, USA) and least sensitive using the Homebrew Simoa assay. Therefore, comparison of assays using sensitivity can be misleading and result in erroneous assay selection. Alternatively, selecting based on which assay can detect the endogenous analyte is more valuable.
Figure 1. Comparison of various sources of IL-13 calibrators in Homebrew Simoa Quanterix assay and IL-13 Simoa Quanterix kit.
Should detectability be a measure of assay sensitivity?
In the absence of interference, detectability is a more accurate measure of sensitivity. Yet, the current perception on assay sensitivity posits that the new, highly sensitive assay platforms, such as Simoa, have enhanced sensitivity over for example, the ELISA format. However, this depends largely on the assay reagents used. In figure 2, we have evaluated two calibrators, human recombinant neurofilament light chain (NF-L) and native bovine NF-L in two different Simoa assays as well as an ELISA assay. The ELISA and the Simoa assay kit from Quanterix use the same reagents compared to the Simoa Homebrew assay, which uses a different pair of antibody reagents. Based on the calibrators, the two Simoa assays are very similar in sensitivity and ~100-fold more sensitive than the ELISA assay [8]. However, when we evaluated the NF-L levels in cerebrospinal fluid (CSF) using the ELISA or the Simoa Homebrew assay, only the ELISA detected NF-L in all CSF samples. This data suggests that the ELISA platform is a more sensitive assay than the Homebrew Simoa for this specific analyte, in spite of the higher calibrator-measured sensitivity. This is due to the specificity of the ELISA reagents for the endogenous NF-L.
Figure 2. A: Comparison of two NF-L calibrators, human recombinant and bovine native NF-L, using Uman ELISA, Homebrew Simoa assay and Quanterix Simoa NFL kit. B: Detection of endogenous NF-L in CSF from four relapsing remitting multiple sclerosis patients using Uman ELISA or the Homebrew Simoa kit.
To get reliable data there is a need for better reagents and evaluation of selectivity/specificity for the biomarker of interest. In addition, evaluation of biomarkers should include appropriate sample size and patient population (patients with appropriate age, gender and ethnicity), appropriate sample collection, processing and storage, as well as taking into account biomarker biological variability, which includes within-individual and between-individual variability as well as longitudinal variability of analytes of interest.
Assay sensitivity based on recombinant calibrators should never be used for determining whether an assay has appropriate sensitivity for use. Instead, detectability of endogenous analyte should guide decision-making. In the last decade, there has been great progress in sensitive assay platform development. However, if not appropriately evaluated, these highly sensitive technologies can produce misleading results by detecting nonspecific signals.
In conclusion, there is significant potential benefits and opportunities for using biomarkers in drug discovery and development as well as clinical management. One key to success is using better assays with characterized reagents specific to the endogenous analyte of interest as well as the elimination of interference in the appropriate matrix for the appropriate patient population.
References
- Drucker E, Krapfenbauer K. Pitfalls and limitations in translation from biomarker discovery to clinical utility in predictive and personalised medicine. EPMA J, 4(1), 7 (2013).
- Poste G. Bring on the biomarkers. Nature, 469(7329), 156−157 (2011).
- Osherovich L. Hedging against academic risk. Science-Business eXchange, 4(15), 1−2 (2011).
- Wagner JA. Overview of biomarkers and surrogate endpoints in drug development. Dis. Markers, 18(2), 41−46 (2002).
- Carden CP, Sarker D, Postel-Vinay S et al. Can molecular biomarker-based patient selection in Phase I trials accelerate anticancer drug development? Drug Discov. Today, 15(3−4), 88−97 (2010).
- Lapointe J, Li C, Higgins JP et al. Gene expression profiling identifies clinically relevant subtypes of prostate cancer. Proc. Natl. Acad. Sci. USA, 101(3), 811−816 (2004).
- Fischer SK, Carrasco-Triguero M, Hong K et al. Commercial biomarker assays: friend and foe. Bioanalysis, 8(22), 2351−2362 (2016).
- Hendricks R, Baker D, Brumm J et al. Establishment of neurofilament light chain Simoa assay in cerebrospinal fluid and blood. Bioanalysis, 11(15), 1405−1418 (2019).
The opinions expressed in this feature are those of the author and do not necessarily reflect the views of Bioanalysis Zone or Future Science Group.