Meet Sei and Orca, the deep learning models used to predict DNA organization and function
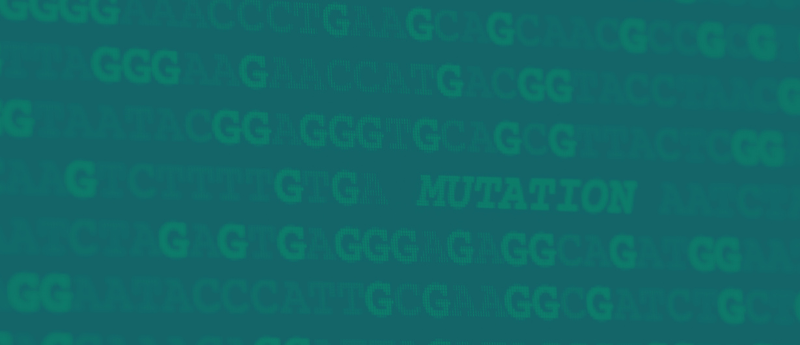
Newly developed artificial intelligence (AI) programs Sei and Orca can predict the function of cis-regulatory elements (CREs), as well as the 3D arrangement of DNA, simply by analyzing variations in a DNA sequence. This research was published across two papers in Nature Genetics and demonstrates the potential use of deep learning models for understanding the mechanisms behind pathogenic mutations.
Genes encoding protein formation make up just 1% of the genome. The remaining 99% houses important regulatory elements, which impact how the coding region is expressed. For example, these elements could be in the form of promotors, which initiate the transcription of genes directly downstream, or silencers, which repress downstream transcription. Although the function of some CREs is known, how sequence composition impacts the function of CREs is not currently well understood.
To help resolve this, a deep learning model called Sei was developed to learn vocabulary relating to 40 regulatory activities, which are termed ‘sequence classes’, and allocate sections of non-coding DNA into these classes. Sei scored the sequences based on predicted activity in each of the classes and could even forecast how mutations affected these activities. This deep learning framework utilized 21,907 publicly available sequence datasets, which covered >97% of the human genome.
You may be interested in:
- eBook: The role of AI in increasing efficiencies in drug discovery and development
- High-accuracy method for identifying disease biomarkers developed by computer scientists
- The business of bioanalysis: new technology integration into bioanalytical workflows
A second study focused on a model named Orca, developed to predict the 3D architecture of DNA in chromosomes based on sequence composition. Existing datasets and structural data from previous studies was used to predict the 3D genome architecture for sequences ranging from a kilobase up to the size of entire chromosomes. Orca could also predict the structure of sequences containing mutations that cause disease phenotypes, specifically a form of leukemia and limb malformations.
Dr Jian Zhou, Assistant Professor at UT Southwestern Medical Centre (TX, USA), and contributing author to both studies, commented:
“Taken together, these two programs provide a more complete picture of how changes in DNA sequence, even in noncoding regions, can have dramatic effects on its spatial organization and function.”
Zhou continued:
“These tools could eventually shed new light on how genetic mutations lead to disease and could lead to new understanding of how genetic sequence influences the spatial organization and function of chromosomal DNA in the nucleus.”
Both deep learning models have publicly available open-source codes accessible via web servers. Together, these models can use sequence data to predict genome organization and CRE function, providing key insights for understanding mutations which lead to human pathologies.
Sources:
Chen KM, Wong AK, Troyanskaya OG, Zhou J. A sequence-based global map of regulatory activity for deciphering human genetics. Nat. Genet. 54, doi: 10.1038/s41588-022-01102-2 (2022), Zhou J. Sequence-based modeling of three-dimensional genome architecture from kilobase to chromosome scale. Nat, Genet, 54, doi: 10.1038/s41588-022-01065-4 (2022), Eureka Press Release, https://www.eurekalert.org/news-releases/961248