Global metabolic profiling – where are we now?
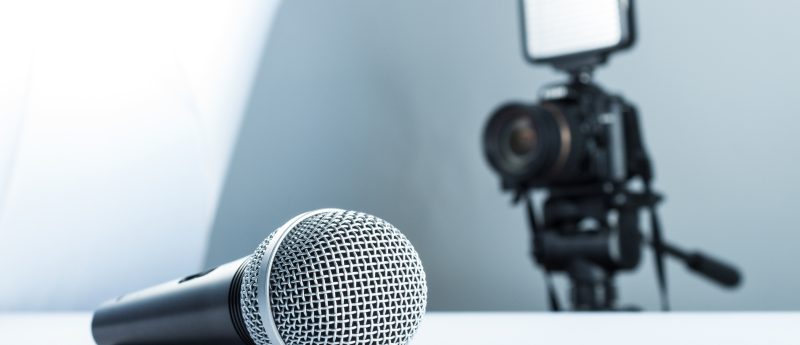
Ian Wilson trained as a biochemist at the University of Manchester, going on to receive a PhD at Keele University. After this, he worked in the Pharmaceutical industry, most recently as a Senior Principal Scientist in the Department of Drug Metabolism and Pharmacokinetics at AstraZeneca, and he joined Imperial College London in 2012. He is the author, or co-author, of some 480 papers or reviews, and has received a number of awards in separation and analytical science from the Royal Society of Chemistry, including the Gold Medal of the Analytical Division (2005) and, most recently, the Knox Medal of the RSC Separation Science Group (2012). He received the Jubilee Medal of the Chromatographic Society in 1994 and gave the inaugural Desty Memorial lecture for Innovation in Separation Science in 1996. His research is directed towards the development of hyphenated techniques in chromatography, and their application to problems in drug metabolism, toxicology and metabonomics.
The metabolome represents an area of increasing focus for systems biologists seeking to understand the complex interactions that take place in biological systems, and also for those who are concerned with trying to find novel, and hopefully mechanistically informative, biomarkers for use in disease diagnosis and monitoring, for example. The requirement for broad, untargeted, metabolic profiling methods designed to cover as much of the metabolome as possible within the constraint of (relatively) high sample throughput, has resulted in the selection of a small number of analytical technologies for this type of work. These are centered on mass spectrometry (MS) and nuclear magnetic resonance (NMR) spectroscopy. MS-based methods include direct infusion (DIMS) or MS hyphenated to a separation system (generally gas or liquid chromatography (GC or LC), but with supercritical fluid chromatography (SFC) and capillary electrophoresis (CE) also options.
There are now a great many publications demonstrating that when combined with rigorous multivariate statistical analysis, these powerful, information-rich, analytical methodologies can and do provide novel biomarkers plus mechanistic insight. The previous is true when presented with samples derived from a properly designed study. Indeed, as a result of developments in this area it is difficult to find an area of biological science where metabonomics/metabolomics is not now finding application. In the area of human biology we are seeing a great expansion of this type of metabolic phenotyping, from small-scale studies (e.g., small-scale clinical trials employing tens to a few hundred subjects in patients) to much larger scale, population-based studies for the metabolic phenotyping (metabotyping) of thousands of individuals. In the case of the former, investigators are generally looking for disease-associated phenotypes (perhaps enabling disease stratification), and metabotypes that will predict therapeutic outcomes. The much larger epidemiological investigations are focused instead on looking for phenotypes that might predict disease risk or that highlight environmental and lifestyle factors (pollutants, nutrition, etc.) where exposure might have long term effects on individuals.
So, if the goal is comprehensive, unbiased, rapid, robust, sensitive and repeatable analytical methodology for metabolic phenotyping (suitable for both small and large scale analysis of the metabolome), where are we in terms of bioanalytical capability? The answer, if we are honest, is probably far enough along the road to be confident that we will discover useful metabolic phenotypes, and from them biomarkers, but by no means at the point where all of the boxes can be ticked. Currently no single analytical platform can be shown to be capable of providing truly comprehensive coverage, not least because just what constitutes the metabolome is still a matter of debate. Any sensible metabotyping program, seeking to maximize its coverage of the metabolome, will therefore need to employ a number of techniques in order to obtain a combined set of data that is as comprehensive as possible.
However, if we take the example of LC-MS, the introduction of UPLC methods increased the metabolite coverage compared to ’conventional’ HPLC throughput increased chromatographic efficiency. In addition, it is well accepted that the widely used ‘traditional’ reversed-phase (RP) separations that formed the backbone of the original LC-based methods work well for medium polar to non-polar metabolites, but not for more polar substances, and were not optimal for lipids. Increasing metabolome coverage and (partially) overcoming the limitations of RPLC for polar compounds was aided by the adoption of hydrophobic interaction liquid chromatography (HILIC) resulting in a significant increase in the coverage of the more polar metabolites, whilst bespoke ‘lipidomic’ methods have greatly improved the analysis of non-polar metabolites. So, perhaps a statement to the effect that “progress has been made, but there is still room for improvement” would be appropriate.
There are obvious differences in the requirements for the stability of the analytical platform used to perform metabolic profiling for small scale studies (10’s to 100’s of samples) versus the larger studies comprising 1000’s of samples and LC-MS-based methods for the latter require rigorous control to obtain sufficient robustness and also ensure that high quality data are obtained.
As for high throughput, again this is a work in progress as, for example, whilst an individual LC-ToF-MS run might only take 10-15min to produce a profile containing thousands of metabolite signals, most likely this is only for one mode of ionization. So if positive and negative electrospray ion data are required then two runs are required on the same sample, and if more than one mode of separation is needed (e.g. RPLC + HILIC), then the overall analysis time might well end up at an hour per sample. Of course the run time could be shortened but this will inevitably result in increased ion suppression and a loss of metabolome coverage. This tension between speed and comprehensiveness of coverage will always result in methods that are a compromise between what the investigators would like to achieve versus what they pragmatically have to do. Despite such considerations, there are many published studies revealing metabolic biomarkers that appear to be highly predictive of the system under investigation.
However, what must be remembered is that these broad-spectrum, untargeted, analytical methods are not really optimized for any of the compounds that they detect (which at that point may still be ‘unknowns’). The metabolic phenotyping studies leading to tentative association of molecules as potential biomarkers thus represent merely the first phase of the ‘biomarker workflow’. The next steps involve the unambiguous identification of these potential biomarkers, followed by the development of suitable specific and quantitative targeted assays for them (or the use of pre-existing validated methods). These methods can then be used to reanalyze the samples to confirm that there is a real association with the molecules and the condition, thereby validating them to the extent that they are at least significant in the test samples. Assuming there is a reasonable biochemical explanation as to why these compounds should be biomarkers, then further studies on different samples are warranted to confirm the connection, and therefore validate the potential utility of the metabolite(s) for future deployment in biomarking the condition.
Overall, significant progress has been made in metabolic phenotyping to the extent that it can be deployed for biomarker detection with a good prospect of success, but there is still a lot to do with regard to method development and optimization, and therefore plenty of room for bioanalytical innovation!
Click here to view our ‘Spotlight on metabolomics’ panel discussion, which Wilson took part in.
Our expert opinion collection provides you with in-depth articles written by authors from across the field of bioanalysis. Our expert opinions are perfect for those wanting a comprehensive, written review of a topic or looking for perspective pieces from our regular contributors.
See an article that catches your eye? Read any of our Expert Opinions for free.