Interview with Adam Rosebrock (University of Toronto) on his metabolomics research
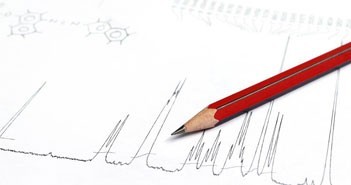
Dr Adam Rosebrock is an Assistant Professor in the Donnelly Centre for Cellular and Biomolecular Research at the University of Toronto, Canada, where he teaches biochemistry, molecular genetics, and genomics. His main research interest is understanding the regulation of gene expression and biochemical activities that underlie cell division and survival across diverse external states. The Rosebrock laboratory actively develops new experimental and analytical methods and builds genetic and computational tools to enable high-throughput and high-content biology, with particular focus on quantitative flow cytometry and MS metabolomics.
Could you tell Bioanalysis Zone a little about your career to date and how you ended up in your current role?
Half a lifetime ago, I started university studies in chemical engineering with a goal of literally creating metabolic pathways. I quickly learned that engineering focused on size of the pipe needed for a given process, and that I am a basic scientist at heart. When I realized how much was yet to be discovered about how cells do the ‘simple’ task of co-ordinately making more cells from simple starting materials, I shifted to molecular genetics and biochemistry.
My graduate training focused on transcriptional analysis, whetting my appetite for big data, after which I pursued a fellowship in functional genomics. I saw huge potential for comprehensive measurement of ‘-omics’ as a way to generate hypotheses that can be tested in the lab, and a place where I could balance basic science with tool building. When setting up my own group at the University of Toronto, I thought carefully about where on the central dogma of ‘DNA->RNA->Protein’ I wanted to focus. I went beyond that map of molecular biology and set out to measure the biochemical activities of the cell. After all, establishing and maintaining metabolic state is what cells evolved to do. The tools of genetics let my group build and break metabolic pathways, while we use LC-MS to directly and quantitatively measure resulting biochemical states. Rather than working to create pathways, I’ve focused on the metabolic complexity that exists in nature.
I understand that the overarching goal of your group is to build a quantitative understanding of cellular metabolism in a ‘cell-state-specific fashion’. What are these ‘states’ and how do you analyze them?
My group is interested in understanding how biochemical processes are (1) linked to cell growth and division, and (2) affected by environmental and nutritional context, including external stresses. Our present methods work best with inputs of thousands of cells; we’re a long way from single-cell metabolomics. Instead of measuring mixed populations, as is commonplace, we use chemical, genetic, and physical techniques to generate homogenous populations of cells. We make extensive use of synchronized cells and time-course experimental designs to characterize transient processes. This approach requires running more samples, but gives us the ability to measure metabolic responses that would be masked in the context of a population average.
Do you carry out un-targeted, full scan, and/or targeted metabolomic studies?
Yes, yes, and yes. Joking aside, we use a range of instruments and methods to best fit the biological question at hand. My group is interested in (1) identifying new biochemical pathways and enzymes and (2) using metabolite levels as a readout of cell state. For discovery or “what’s different between n samples?” questions, we start with our ‘Jack-of-all-trades’ chromatography and untargeted analysis on full scan qTOF instruments. Our next steps often involve placing a full-scan instrument behind an orthogonal or analyte-optimized chromatography to follow up on interesting features (see below). For known metabolites and for new compounds we’re identifying, we build targeted analyses for use on the triple-quadrupole. This approach enables complementary analytical breadth (full scan) and depth (targeted). We have projects on the QqQ where we’re examining a dozen analytes across a few thousand samples, but we came to those target analytes after extensive full-scan, untargeted analysis.
You mentioned that you use un-targeted, full scan, MS in hypothesis generation. How is this technique employed to create an experimental hypothesis?
In many big data contexts, ‘hypothesis generation’ is used as a polite synonym for ‘fishing expedition’. In LC/MS metabolomics, untargeted full scan data acquisition is the first step in what’s often a longer analytical process. In a well-designed experiment, many analytes don’t change. This is a good thing. We use full-scan analysis to generate a list of mass spectral features that appear to change as a function of the experiment. This list of experimentally-covariate features is, in essence, a set of immediately testable hypotheses.
Once your hypothesis has been developed, how do you identify experimentally-responsive analytes?
The old adage about getting the answer you ask for holds true – these are high-dimensional data, and it’s easy to find differences between groups of samples, even if it is just by chance. We often generate an orthogonal sample set and ask “are the observed changes consistent across different days/hands/animals/reagent lots?” Much of our work harnesses the power of reverse genetics, so we test independent mutants, different shRNAs, or independent genetic backgrounds. In cases where generating more samples isn’t feasible, we use a cross-validation approach to analyse replicates.
What are the processes involved in development of targeted assays for hypothesis testing and compound identification?
With a robust list of candidate changes in hand, it’s back to the bench. Follow-up experiments vary, and include collision induced fragmentation for compound ID and a first-pass deconvolution of co-eluting isobars, use of orthogonal or shallower gradient chromatographies, stable isotope labelling, and eventually compound verification and semi-quantitation by standard spike-ins. With current high-sensitivity instruments, samples we collect often provide sufficient material for the multiple injections needed. The same LC/MS instruments, Agilent UPLC/qTOFs in my group, are capable of running every step of these analyses. One of the great powers of LC/MS metabolomics is flexibility of the instruments. We move to targeted assays on a QqQ for sensitivity and throughout. One of the strengths of Agilent’s LC-MS offering is the use of many parts of the hardware and software in common across qTOF and QqQ instruments, making it relatively easy to migrate methods from full scan to targeted analysis.
Tool building plays a big role in your research. What is the most innovative tool your research group has produced and what for what purpose was it necessary?
I think the most useful tool we’ve recently developed isn’t our most innovative, but has nevertheless been transformative. A recurring problem in full-scan metabolomics is distinguishing experimentally-derived features (made by the cells under study) from media contaminants and solvent peaks. With a significantly changing metabolite in hand, one of the first questions we ask is “what’s the empirical formula?”
We solved both of these problems with a well-designed experimental framework and our custom software to build a ‘white list’ of biological compounds with associated carbon and nitrogen atom counts. We start by growing cells on 13C, 15N, or double labelled chemically-defined media where all carbon or nitrogen is heavy isotope. We harvest after >10 generations, when labelled nutrient makes up 99.9% of culture-derived metabolites. Contaminants of all sorts will remain unlabelled. Using software written in the lab, we are able to (1) identify ‘biological’ compounds as those which shift in either or both heavy-isotope experiments, and (2) generate C and N counts for each feature. This approach lets us quickly triage candidate peaks as biological or contaminant, and, combined with formula prediction from isotopic spacing and accurate mass, gives us a head start on compound identification.
Do you believe that the development of a comprehensive metabolomics multiple-reaction monitoring (MRM) library and methodology is necessary?
Absolutely! There is enormous potential in LC/MS metabolomics, but many labs are stymied by the significant technical hurdles of assay development. Although I’ve focused on full-scan, untargeted approaches today, measuring a set of known metabolites is sufficient for many research questions. We’re actively developing a portable set of robust chromatographic methods and characteristic transition libraries to enable other labs to quickly adopt metabolomics as part of their workflow. I look forward to the inflection point when the metabolomics community shifts focus from assay development toward generating samples and collecting great data. After all, my group builds tools as part of our efforts to understand biology.
How do you believe our knowledge of metabolism has progressed over the years?
The biochemical wall-charts that adorn lab hallways represent knowledge collected across a range of organisms and tissues ranging from batch-fed E. coli to beef heart. This ‘slaughterhouse biochemistry’ of the last century provided a wealth of information about the activities that life can perform, but falls short of describing how organisms with different genetic complements maintain homeostasis when faced with different intra- and extracellular signals. Metabolism is tissue, cell-type, and extracellular context specific. We now have the tools to move beyond textbook summaries of metabolism to understand how to increase efficiency in bio processing, identify biochemical Achilles’ heels of pathogens, and determine how tumours are biochemically different from non-transformed tissues.
What are you excited about working on over the next year?
Using full-scan LC-MS, we’ve recently identified a few dozen new enzymes as part of a genetics-driven metabolomic screen performed in close collaboration with Amy Caudy’s group here at the University of Toronto. With the formulae of product and precursor metabolites of our new enzymes in hand (see above!), we’re moving on to determination of chemical structure of the new metabolites. From there, we’re developing targeted MRM assays for these new compounds to use LC-MS as a readout for enzyme kinetic measurements and as a tool to determine of the role of these enzymes across different biological contexts. In the next year, we’ll put names and functions on as-yet uncharacterized genes and potentially re-write the textbook view of a few pathways along the way!
Click here to view our ‘Spotlight on metabolomics’ panel discussion, which Rosebrock took part in.